COVID-19
COVID-19 was first identified in December 2019 and was declared a global pandemic within months. It became the second-leading cause of death in 2021, causing a global decline in life expectancy.
Photo by Reuters/Alisha Jucevic.
How often do we produce COVID-19 estimates?
From 2020 to 2022, we produced regular estimates of cases, hospitalizations, and deaths from COVID-19, as well as 4-month forecasts of trends in the pandemic. We updated our projections frequently as new data became available and responses to the pandemic evolved, for example beginning to incorporate the use of vaccines.
Our forecasting model was designed to be a planning tool for government officials who needed to know how different policy decisions could radically alter the trajectory of COVID-19 for better or worse.
In December 2022, we paused our COVID-19 modeling and began including total cases, deaths, and disability from COVID-19 in the Global Burden of Disease (GBD) study.
What modeling approach did we use for COVID-19 forecasting?
We used a hybrid modeling approach to generate forecasts, which incorporated elements of statistical and disease transmission models. Our model was grounded primarily in real-time data, and we updated it frequently to respond to new data and new information.
At two points, we made major updates to our modeling approach to account for:
- Total and excess mortality, including unreported deaths
- The Omicron variant and waning immunity from infections or vaccines
Our model:
- Showed how different policy decisions could impact the trajectory of COVID-19
- Incorporated data on deaths, hospitalizations, and cases adjusted for scale-ups in testing and populations tested (i.e., symptomatic individuals and active case detection efforts among high-risk populations in factories, prisons, nursing homes, and homeless shelters)
- Corrected for errors in reported data
- Considered both reported COVID-19 deaths and total COVID-19 deaths in each population
- Factored in important drivers of trends in COVID-19, such as vaccination rates, mobility, population density, testing, self-reported mask use, seasonal patterns of pneumonia (these patterns closely mirror transmission of COVID-19), and self-reported contacts to understand transmission of the virus
- Relied primarily on real-world data
- Took into account variation in transmission across locations and over time
- Made sense of data that fluctuated frequently and new findings across the globe in real time
What data did we use for COVID-19 forecasting?
Our forecasts included data from a range of sources, including:
- Local and national governments
- Hospital networks and associations
- The World Health Organization
- Third-party aggregators
Data on reported death numbers
For some locations, we used the reported death numbers, with the vast majority of these coming from the Johns Hopkins University (JHU) data repository on GitHub, to collate daily COVID-19 cases and deaths.
We supplemented this dataset as needed to improve the accuracy of our projections. For example, we used data from government websites for a number of locations and for subnational estimates.
Data on testing
Our primary source for US testing data was the US Department of Health and Human Services, through the HHS Protect Public Data Hub.
For other global locations, we used primarily what was reported by Our World in Data (OWiD), supplemented by location-specific information typically sourced from government agencies, should such data be absent from the OWiD database.
Data on total infections
We used serosurvey data that evaluated the antibody-positivity of the population sampled, in order to better determine the total number of infections that are present among the population.
These data were sourced from a variety of locations, but a significant proportion were sourced from SeroTracker, an open repository of published serosurvey datasets, in addition to ongoing state-sponsored serosurveys that occurred at weekly or monthly frequencies, such as the US CDC’s blood donor survey.
Data on hospital resource use
We obtained hospital resource data from sources such as:
- Government websites
- Hospital associations
- The Organisation for Economic Co-operation and Development
- The World Health Organization
- Published studies
Data on mobility and population density
For population density, we used gridded population count estimates for 2020 at the 1 x 1 kilometer (km) level from WorldPop.
For mobility, we used anonymized, aggregated data from Google.
Data on mask use
Our mask use data sources were:
- Premise (US only)
- The Delphi Group at Carnegie Mellon University and University of Maryland COVID-19 Trends and Impact Surveys, in partnership with Facebook
- Kaiser Family Foundation
- YouGov COVID-19 Behaviour Tracker survey
Data on vaccines
We obtained data on vaccine supply from Linksbridge, and data on vaccine hesitancy from a Facebook survey jointly conducted with MIT.
Data on vaccine administration were primarily sourced from Our World in Data, supplemented by location-specific information typically sourced from government agencies, should such data be absent from the OWiD database.
In particular, we used local datasets to obtain age-stratified and brand-specific distribution statistics.
Data on excess mortality
Excess mortality data sources used in our estimation of total and excess mortality due to COVID-19 are available via this downloadable file.
We would also like to thank the GISAID Initiative and are grateful to all of the data contributors, i.e., the authors, the originating laboratories responsible for obtaining the specimens, and the submitting laboratories for generating the genetic sequence and metadata and sharing via the GISAID Initiative, on which this research is based. GISAID data provided on this website are subject to GISAID’s Terms and Conditions. Individuals and their contributing laboratories are outlined in full at CoV-Lineages.
Read more about GISAID’s contributions to global health
What do the different scenarios mean?
We included various scenarios at different points in the pandemic to reflect the priorities of the time and new developments. For example, our initial forecasts focused on hospital capacity and non-pharmaceutical interventions like social distancing mandates, while our most recent ones included the availability of vaccines and antivirals.
In our last forecast, we produced these three scenarios:
The reference scenario is our forecast of what we think is most likely to happen:
- Vaccines are distributed at the expected pace. Brand- and variant-specific vaccine efficacy is updated using the latest available information from peer-reviewed publications and other reports.
- Future mask use declines to 50% of the minimum level it reached between January 1, 2021, and May 1, 2022. This decline begins after the last observed data point in each location and transitions linearly to the minimum over a period of six weeks.
- Mobility increases as vaccine coverage increases.
- Mandates are reimposed at the maximum level of mandates in the post-ancestral period once the death rate has reached an algorithmic minimum threshold of daily reported deaths for a given location.
- 80% of those who are fully vaccinated (two doses for most vaccines, or one dose for Johnson & Johnson) receive an additional dose six months after becoming fully vaccinated, and 80% of those who receive an additional dose receive a second additional dose six months later.
- Antiviral utilization for COVID-19 risk prevention has reached 80% in high-risk populations and 50% in low-risk populations between March 1, 2022, and June 1, 2022. This applies in high-income countries, but not low- and middle-income countries, and this rollout assumption follows a similar pattern to global vaccine rollouts.
The 80% mask use scenario makes all the same assumptions as the reference scenario but assumes all locations reach 80% mask use within seven days. If a location currently has higher than 80% use, mask use remains at the current level.
The antiviral access scenario makes all the same assumptions as the reference scenario but assumes globally distributed antivirals and extends coverage to all low- and middle-income countries between August 15, 2022, and September 15, 2022.
Why are the “reported” deaths shown in our results different from what is shown on the government’s official page?
We obtained deaths data from a variety of sources. For some locations, we used the reported death numbers, with the vast majority of these coming from the Johns Hopkins University (JHU) data repository.
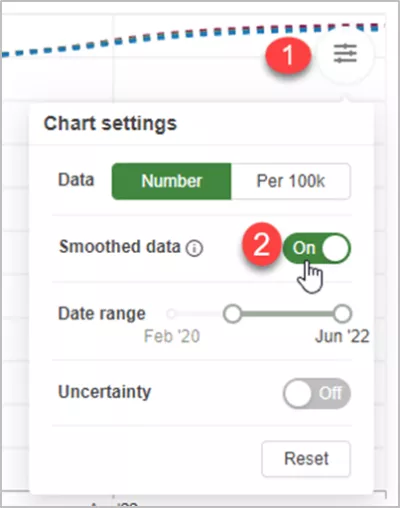
Given that reported numbers were subject to frequent revision, often impacting the entire history of the pandemic, where substantial revisions occurred and death data were temporally indexed by “day of death,” we used that time series instead.
Finally, for some locations, such as Mexico and Russia, where periodic cause of death data were released, we scaled reported death numbers to match the final cause of death database releases. Cause of death data were usually more complete than the releases from surveillance systems. However, the trade-off is that they were released several months after the fact.
We also estimated the fraction of excess mortality in each country that was directly related to COVID-19 and the fraction that was increased mortality in individuals who did not test positive for COVID via PCR testing at the time of death. Please see our Estimation of total and excess mortality due to COVID-19 page for further details.
Yet another reason why observed deaths may differ from numbers reported by governments was due to data processing. To address irregularities in the daily death data, we averaged model results over the last seven days to create a smooth version. To see the death data exactly as it was reported, click the “chart settings” icon in the upper right corner of the chart and turn off “smoothed data.”
How were vaccines incorporated into the model?
We updated brand- and variant-specific vaccine efficacy using the latest available information from peer-reviewed publications and other reports. For more information on the assumptions about vaccine efficacy that we used in our models, see our COVID-19 vaccine efficacy summary.
We also incorporated vaccine hesitancy, available dose, estimation of people vaccinated, brand distribution, and boosters into our model.
How was hospital resource use incorporated into the model?
The hospital resources shown are those we estimated were available for COVID-19 patients. We have excluded non-COVID patient needs, that is, the typical percentage of hospital beds occupied by other patients and emergencies.
Our estimates changed as new data came in. Specifically, new death data and new information about the number of COVID-19 patients who need hospital beds changed our projections.
Discrepancies between our projections and other data dashboards typically stem from the limitations of the datasets that we used to estimate hospital and ICU beds needed for COVID-19 patients. We did not have access to data that reflected how bed counts were changing in real time. Note public records of the number of hospitalizations on a particular day did not account for the number of people who are already occupying beds.
How did we estimate infections?
We defined estimated infections as prevalent infections – that is, all cases that exist in a location on a given day, not just new ones. Confirmed infections were those infections that had been identified through testing.
We estimated past daily infections in a modeling framework that leveraged data from seroprevalence surveys, daily cases, daily deaths, and, where available, daily hospitalizations.
We incorporated several factors as drivers of infections:
• Increases in human mobility
• Loosening of social distancing measures
• Seasonal disease transmission patterns
• Declining vigilance (mask use declining and human contact increasing)
• Emergence of new variants
• Lower vaccination rates
Read more about how we estimated infections in our peer-reviewed articles:
Acknowledgments
We wish to warmly acknowledge the support of these and others who have made our COVID-19 estimation efforts possible.
- ACAPS
- American Heart Association
- American Hospital Association
- Bill & Melinda Gates Foundation
- Blavatnik School of Government, University of Oxford
- Bloomberg Philanthropies
- Boston Children’s/Health Map
- California Health Care Foundation
- Carnegie Mellon University
- Centro de Investigaciones en Ciencias de la Salud, Universidad Anáhuac
- Department of Political Science, University of Washington
- Descartes Labs
- Facebook Data for Good
- Fundación Mexicana para la Salud
- GDS Services International: Tómatelo a Pecho A.C.
- GISAID Initiative
- Google Labs
- John Stanton & Theresa Gillespie
- Julie & Erik Nordstrom
- Kaiser Family Foundation
- Medtronic Foundation
- Microsoft AI for Health
- National Institute on Minority Health and Health Disparities (NIMHD) at the National Institutes of Health (NIH)
- National Science Foundation
- Our World in Data
- Premise
- Qumulo
- Real Time Medical Systems
- Redapt
- SafeGraph
- The COVID Tracking Project
- The Johns Hopkins University
- The Kuwait Foundation for the Advancement of Sciences (KFAS)
- The New York Times
- UNESCO
- University of Maryland
- University of Miami Institute for Advanced Study of the Americas (Felicia Knaul, Michael Touchton, and Héctor Arreola-Ornela)
- US Department of Health and Human Services
- Wellcome Trust
- World Health Organization
- And finally, the many Ministries of Health and Public Health Departments across the world, collaborators, and partners for their tireless data collection efforts
Thank you